Having food is an immediate primal necessity for everyone. Risks to food security are therefore of utmost importance and can be caused by numerous factors, such as a growing population, increasing meat consumption with its associated requirements for crop land, and climate change.
Various, opposing mechanisms can be at play in this topic. A changing climate with different temperatures and changed precipitation regimes could be either advantageous or disadvantageous for crop growth, depending on the crop characteristics. On the other hand, an increased CO2 concentration in the atmosphere has a fertilization effect for plants, thus increasing crop yields.
Materials and methods
To investigate changes in crop yields due to climate change in quantitative terms, we analyzed model results that simulated historical as well as projected agricultural yields. Here we look specifically at the occurrence of crop failures. A further goal was to examine how climate change that has already occurred affected crop failures so far.
To do so, we looked at the output of three global crop models (i.e., GEPIC, LPJmL and PEPIC). These models simulate crop growth for given inputs, such as crop type, soil type, weather and fertilization. All simulations were carried out in the context of the ISIMIP project. In this analysis we used data obtained in round ISIMIP2b, which is driven by climate data following a low emission scenario (RCP2.6) and higher-emission scenario (RCP6.0) greenhouse gas scenarios.
We looked at the output variable yield, measured in tons per hectare, which provides potential yields, both for purely rainfed and for fully irrigated agriculture. Potential yields means that the models simulate the planting of crops at every place on Earth and then calculate the potential yield for each grid cell. For this investigation we looked at the four major crops: corn (maize), rice, soy, and wheat. To obtain actual production numbers, we need to determine which areas are actually used for crop farming. The ISIMIP project provides crop lands for rainfed agriculture and for fully irrigated agriculture. The ISIMIP land use data covers the historic period as well as projections for the futures under the two mentioned greenhouse gas scenarios (RCP2.6 and RCP6.0). These projected future land use scenarios are specific to the climate model (i.e., GFDL-ESM2M, IPSL-CM5A-LR, HadGEM2-ES, MIROC5), but assume socioeconomic conditions of an SSP2 world. For details on these land use data please refer to the ISIMIP2b protocol. In this analysis Greenland and Antarctica are ignored.
As stated earlier, we also want to look at the effects of the climate change that has already occurred. Therefore crop failures were defined relative to the preindustrial climate, i.e. the climate without anthropogenic climate change. We simulated the potential yields of the four crops for the pre-industrial period. From this run we then determined the 2.5th percentile at each location and use this value as the threshold for a crop failure. If at any time the hindcasted/projected crop yield falls below that threshold, it is considered a crop failure.
To determine the total land area exposed to crop failure, we add up the crop areas where the crop failure occurs. Besides, we also investigated the share of the population exposed to these extreme events. In order to accomplish this ISIMIP2b population data was used, and the number of exposed people is essentially determined from the number of people employed in agriculture. While we used actual population data for the historic time up to 2005, the population was held constant at 2005 levels for all years after 2005. This allows us to isolate the climate change effect on the exposure.
Further details about all these definitions are given in Lange et al. (2020).
Results
Global averages
Compared to pre-industrial times the global mean temperature is currently about 1 °C higher. According to the projections, even the climate change experienced so far caused an increase in the land area exposed to crop failures (Figure 1). While in pre-industrial times about 0.1 % of the land area (without Greenland and Antarctica) were exposed to crop failure, this share has risen to 0.15% in a 1 °C world. For the other warming levels the total land areas exposed are projected to be 0.11% at 0.5 °C, 0.2% at 1.5 °C and 0.24% at 2 °C. 2 °C is the global warming all climate models reached, but some projected even hotter temperatures. For IPSL-CM5A-LR data is available up to +3.5 °C. This difference in data availability occurs because some models simulated longer time frames up to 2299, which increases the likelihood of reaching these higher warming levels.
Explore how land and population exposure to crop failure vary with global warming and time at the global and national level
Show exposure of
Show how exposure varies with
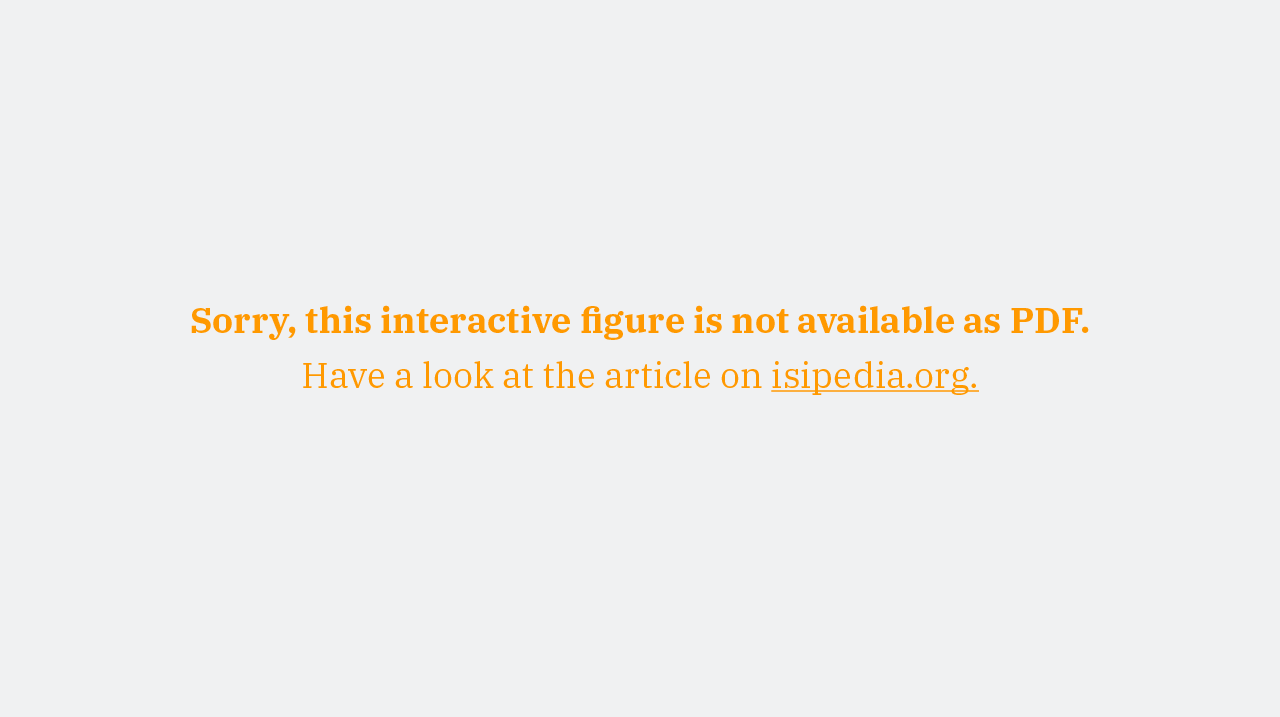
Click on any dot to see a disaggregation of the global results to the national level. Compare results obtained with different models by selecting All in the drop-down menus or by clicking on their headers. Use the toggles to change what is represented by the axes of the line plot. The NDC line represents the expected global warming level for the given year assuming the mean of the unconditional worst, unconditional best, and conditional NDC pathways. See this article for more info. Use the Download Data button to get numeric results for all model combinations.
FIG 1 / Adapted from Lange et al. (2020).
Due to the inhomogeneous distribution of people across the globe the numbers for the exposed population deviate from the numbers for the land surface exposure. Globally, we see 0.59% of the global population exposed to crop failures in a pre-industrial world. Especially at higher warming levels this number increases. At the current +1 °C conditions we see 0.6% of the people exposed. For the other warming levels the projections are 0.56% at +0.5 °C, at +1.5 °C this rises to 0.82%, while at +2 °C 1.02% of the population are projected to be exposed to crop failure. Note that the differences between the individual model runs can be quite sizable, and thus uncertainty can be too. Especially the simulations by the IPSL-CM5A-LR climate model project larger exposures than the other models (0 °C: 0.10%/0.45%, 0.5 °C: 0.19%/0.60%, 1.0 °C: 0.27%/0.81%, 1.5 °C: 0.32%/1.1%, 2 °C: 0.34%/1.4%).
Figure 1 allows you to explore these details on your own. The top switch lets you pick whether to look at the exposed land area or the exposed population shares. You can select the mean across all climate and impact model simulations, or model specific data. As briefly mentioned above, individual model runs might disagree, a fact that is obscured by just giving the mean across all model runs. Playing with these settings is a way to get a feel for the uncertainties involved.
So far we only discussed exposures at different warming levels. However, these were derived from timelines, which is the actual model output (select “Time” in Figure 1). Be aware that even though the climate models project climate data as a timeline, the exact timeline is meaningless. All we can look at is statistics across sufficiently large time slices, typically 30 years. Thus, we cannot say that on the 2nd of February 2043 the mean temperature will be at a particular location 17 °C, but only that the mean temperature of 2050 to 2079 at that location is, say, 14 °C. In this analysis we chose 20 years for the duration of a time slice. When you look at the results given for 2010, you really look at the mean values of 2001 to 2020.
Results at the national level
So far we only presented globally averaged exposures, but the next plots show results at the national level. Figure 2 shows for each country the exposed land area/population (pick “Annual exposure”) or the ranking compared to all other countries (pick “Exposure ranking”). For instance, when looking at the map of land exposure for 2 °C, one can see that the Indian subcontinent (both Pakistan and India) is most highly exposed. Other countries with a rather large share of exposed land can be found in North America, Europe and also in West Africa. Looking at the exposed population still shows a high exposure in Pakistan and India, but China, Mexico, Egypt and several countries in Africa also emerge as countries with rather highly exposed population shares.
Explore how land and population exposure to crop failure at 2°C global warming vary from country to country
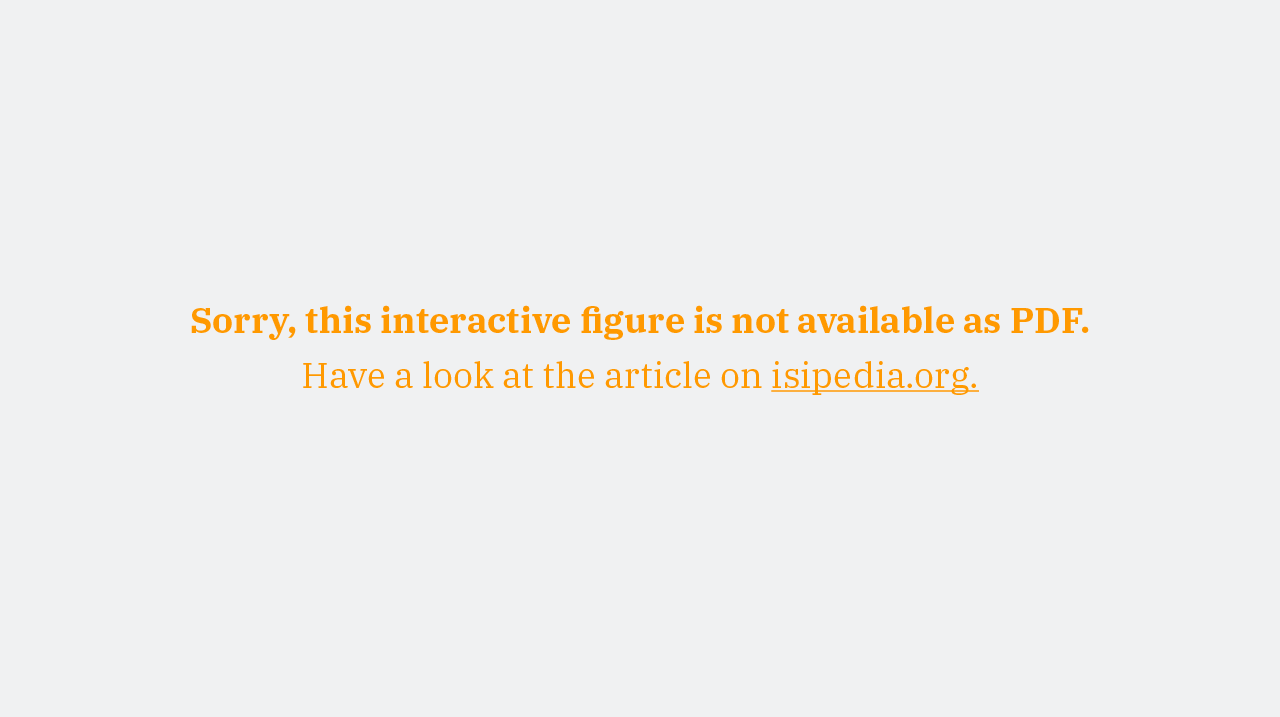
Use the toggles to switch from a country ranking by exposure to annual average exposure values and from land exposure to population exposure. Use the Download Data button to get numeric results for the current view.
FIG 2 / Adapted from Lange et al. (2020).
When looking at the rankings, we find that, at 2 °C global warming, Pakistan is projected to be the country that is most exposed to crop failures, both in terms of land area (2.4 %) and in terms of population (4.6 %). This means that almost 1 in 20 people is exposed to a crop failure events on average in a given year. The other countries with high exposed land areas are 2nd India (1.4 %), 3rd Iraq (1.3%), 4th Morocco (1.2 %) and 5th El Salvador (1.1 %). In terms of exposed population in a 2 °C world we find 2nd Nepal (3.8%), 3rd Iraq (3.5%), 4th Egypt (3.0%) and 5th Morocco (2.4 %).
Limitations
In the introduction we hinted at the fact that a multitude of processes determine the intensities and frequencies of crop failures in an intricate interplay. A major process in this is the CO2 fertilization effect, an effect which is not yet as precisely quantifiable as we would like it to be. This is a systematic uncertainty that still requires more research. Another uncertainty derives from our limited understanding of the greenhouse gas effect. For instance, in CMIP6, in order to reach the same increase in radiative forcing for a given RCP, more CO2 than in CMIP5 is assumed to be in the atmosphere. This has contributed to changed yield projections for the same RCPs between CMIP5 and CMIP6 driven agriculture simulations. Another source of uncertainty are the crop areas. While we have “observations” for the crop areas for the past, for the future we must rely on projections that make a multitude of assumptions. The crop land area projections need to assume future population numbers (a discrepancy to our fixed population in the future), market prices, technological advances etc.
Apart from these sector specific uncertainties the usual modelling uncertainties come into play. We have uncertainties in the climate models, as well as in the impact models. They differ in the processes they implement, their relative importance and so on. Also input data, such as soil maps, are not always 100% correct.
Nevertheless, the basic statement that an increased warming causes increased exposures to crop failures is supported by all models.
Acknowledgements
This summary article was written in collaboration with the ISIpedia Editorial Team.
Contact
Please contact the ISIpedia Editorial Team (isipedia.editorial.team@pik-potsdam.de) for more information or questions about this report.
References
Cover image: Lena Scheiffele
Affiliations
1 Potsdam Institute for Climate Impact Research, Member of the Leibniz Association, Potsdam, Germany
2 School of Geographic Sciences, East China Normal University, Shanghai, China